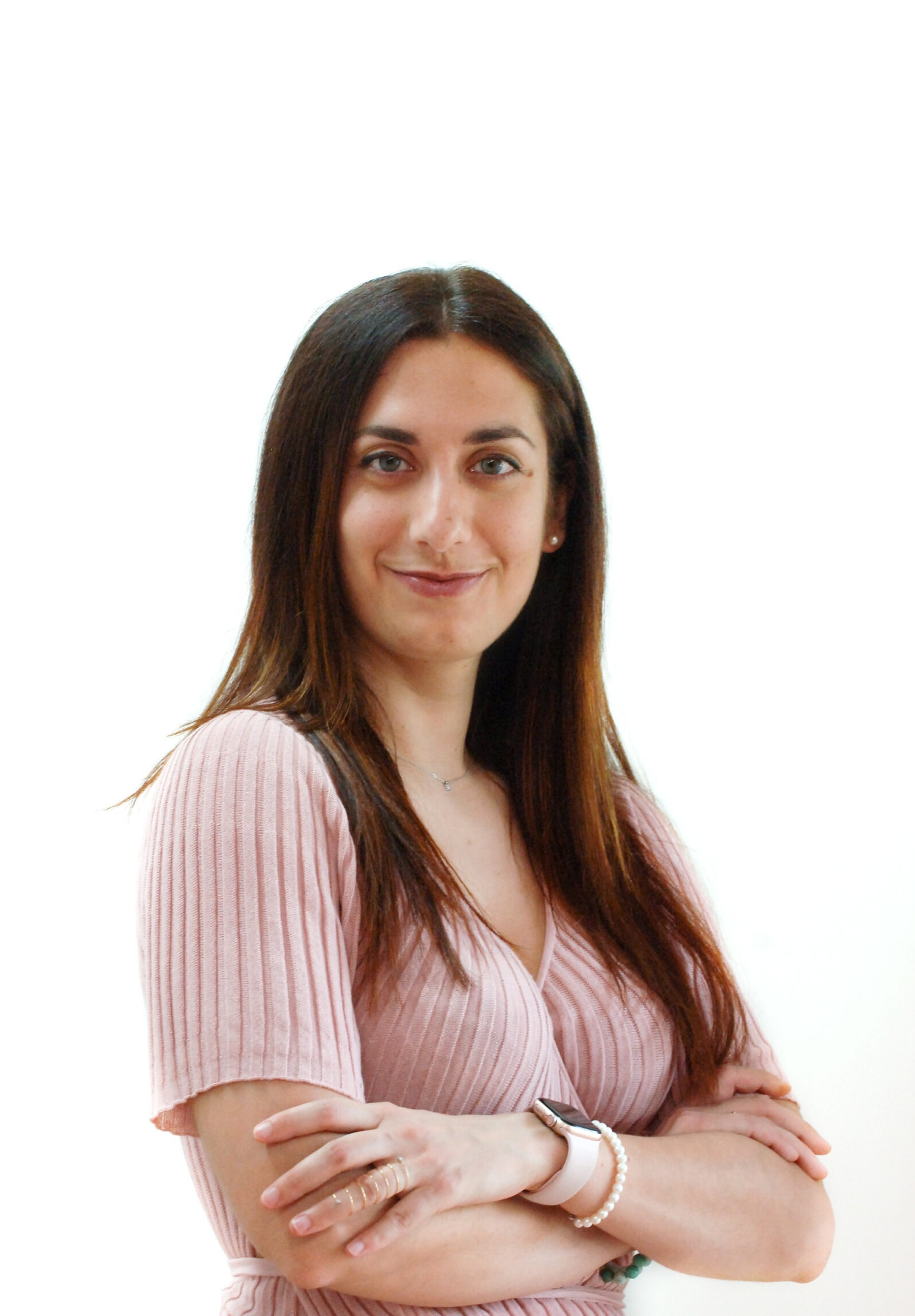
I am Junior Assistant Professor in Numerical Analysis at the MOX (Modeling and Scientific Computing) Lab of the Department of Mathematics, Politecnico di Milano.
I hold a Master degree in Mathematical Engineering, with a specialization in “Computational Science and Engineering”, from Politecnico di Milano. During the MSc, I spent six months as exchange student at Sorbonne Université in Paris. This period was an invaluable experience that significantly broadened my academic and personal horizons. After the graduation, I started an internship in the risk advisory team at Ernst&Young, one of the consulting firms among the “big four”. In November 2017, I started a Ph.D. project in “Mathematical Models and Methods in Engineering” at MOX, within the European Research Council (ERC) Advanced Grant Project iHEART, under the supervision of Prof. Alfio Quarteroni. Since then, my research activity focused on computational reduction techniques, or reduced order models (ROMs), for solving complex differential problems, depending on several parameters related to physical and geometrical properties of the system. Indeed, there is a pressing need for fast and ecological alternatives to a massive usage of HPC resources to compute the solution of high-fidelity (e.g. finite element) models.
In particular, I developed a new class of non-intrusive nonlinear ROMs based on deep learning (DL) algorithms, called DL-ROMs, which have shown the ability to perform real-time solution computations in milliseconds, a significant improvement compared to the hours or days needed by standard numerical methods. These models have been applied to several complex problems in fluid dynamics and its interaction with solid mechanics, cardiac electrophysiology, cardiac mechanics and Micro-Electro-Mechanical Systems (MEMS) applications.
My ongoing research centers on scientific machine learning, with a primary focus on deep learning-based surrogate modeling and neural network approximation theory. Specifically, I use deep learning algorithms to create surrogate models that accurately and efficiently approximate the behavior of complex systems, while focusing on their mathematical foundation and justification.
I remember that when the first papers on scientific machine learning appeared in 2017, they were met with considerable skepticism within the scientific community. This skepticism stemmed largely from the fact that the proposed methods, which relied on 'black-box' neural networks, lacked a rigorous mathematical justification. Traditionally, the path in numerical analysis has been to first establish mathematical theory, including error estimates, convergence, and stability analysis, before applying these methods to real-world problems. However, in the case of scientific machine learning, the approach was inverted: researchers began applying deep learning algorithms across various domains, yielding impressive results, before focusing on the underlying approximation theory. It is important to note that neural networks do have a (very complex) mathematical foundation; it's just that this foundation is currently being explored.
Seven years after coming back to university to start my Ph.D., I am happy I made this choice since research allows me to express my curiosity about what I do not know, to use my creativity in identifying a solution to unsolved problems, and thus to contribute to the advancement of knowledge and technological innovation. A natural curiosity for innovative approaches, the capacity to disseminate one's work and findings, and the aptitude for collaboration, along with ambition and dedication to real-world problems, uniquely qualify a researcher to make a meaningful contribution in reaching the goal of proposing novel computational methods with incredible potential for direct societal impact.
My advice for students who are about to complete their degrees? During your studies, endeavor to discover your true passions and topics of proficiency. Seek out the areas where you might lose in without realizing that time is moving forward. University education shouldn't be a race against time; rather, it should foster not only the establishment of a robust background for embarking on your professional journeys but also resourcefulness, critical and out-of-the-box thinking, stress management, and the capacity for independent work. Moreover, for young female students, I would like to add a personal note. As a female researcher in STEM, I cannot deny that gender imbalance in these fields remains a pressing issue, with women significantly underrepresented in various sectors. In addition to the intrinsic difficulties characterizing technical roles, women also encounter the inevitable obstacle of living in a world that often struggles to accept their affirmation. I would like to instill a message of courage, determination, and strength regarding job positions that may appear complex and demanding for women. I want to encourage perseverance and highlight the importance of skills and knowledge as the key ingredients for building a career based on merit, not gender. Abilities and efforts will pave the way for success, and perseverance will help break down the barriers that still exist.